Who will provide AI to the world?
The third post in our series On AI Deployment (By Aspen Hopkins, Andrew Ilyas, Aleksander Madry, Isabella Struckman, and Luis Videgaray)
Last time, we discussed the structure and complexity of AI supply chains. We unpacked why this complexity is different from previous supply chains and how it challenges existing priorities in AI policies such as accountability. In this third segment of our series On AI Deployment, we consider the economic ramifications of upstream–specifically base–models. We ask how market concentration upstream of the AI supply chain might (or might not) occur, and outline the likely futures that either scenario could lead to.
On March 14, 2023 (“Pi Day”), three prominent developers of generative AI showcased their latest products. Google revealed it is now integrating chatbot capabilities into its Workspace apps (including Gmail and Docs); Anthropic announced a rollout of its new large language model Claude; and OpenAI revealed its highly anticipated new multi-modal large model, GPT-4. The fact that these announcements occurred on the same day illustrates the intensity of the competition in the field of generative AI1. And even before the “Pi Day” announcements, there was already a surge of venture capital interest in generative AI.
Amidst this whirlwind of AI euphoria, critical questions regarding the technology's implications remain unanswered. Some of these questions stem from our direct interaction with these novel AI tools: how will they change our cognition, our creativity, and the very fabric of our social relationships? Other questions concern the broader social and economic ramifications of AI, such as the impact on labor markets or intellectual property rights.
Today, we dissect another question, one that will be pivotal for both business strategy and economic policy: will the global supply of base (or foundation) models be monopolized by a handful of titans, or will it flourish in a competitive market structure?
In other words, who will hold the reins as AI shapes the world?
In this post, we’ll explore two possible futures of base AI models: healthy competition (where there are a variety of AI providers—good for users), and market concentration (where only a handful of “big players” provide AI models—bad for users). We consider three factors that affect the likelihood of the latter, namely scale effects, data network effects, and platform effects.
Two Futures
As base AI models are poised to become an important component of the global economy, the evolution of their market structure will be vital to companies, investors, policymakers, and consumers. However, a future where only a few dominant players control the market looks dramatically different from one where many developers engage in intense competition.
Healthy Competition
Let’s first consider the ideal–a market for base models with healthy competition between diverse actors. In this scenario, we expect downward pressure on the prices that downstream developers and end users are charged. Upstream actors are held to a competitive standard, reducing the possibility of rapid vertical integration2 or harmful price coordination. It’s certainly possible that in such a climate, resource-intensive base models become a low-margin commodity, as some propose. Or, instead, perhaps the competitive environment will lead to “numerous, high-utility AI systems … [that] emerge, distinct from [single] general AI models”3.
Market Concentration
In contrast, if the supply of base models is concentrated in the hands of only a handful of upstream suppliers, we may observe the consequences of oligopolistic or monopolistic behavior: price inflation, artificial availability or access limits, and an accepted norm of poorer-quality offerings. This is suboptimal for both consumers and downstream actors.
Beyond anti-competition, concentration can lead to concerns regarding reliability. First, if a sizable portion of society or an industry is dependent on only a small number of base models (controlled by two or three companies), what happens if one model malfunctions? A single failure can have disastrous downstream consequences. Second, when these failures do happen (whether they correspond to outright outages or just undesirable behavior), market concentration means that the free market will do little to compel base model developers to restore functionality quickly.
In short: market concentration is never good for users. Knowing this, what should we pay attention to in order to reduce its likelihood?
Possible Drivers of Market Concentration
For the remainder of this post, we focus on three historically relevant sources of persistent, technologically-driven market concentration: scale effects, network effects, and platform effects. We discuss how each of these effects may (or may not) present themselves in upstream AI products. The mere possibility of these effects arising suggests that AI market concentration is a genuine concern that should not be underestimated by analysts and policymakers.
Driver I: Scale Effects
The first potential driver of market concentration of AI is the ever-improving returns from scaling machine learning models and datasets.
Scale has had a profound impact on AI technology. The technical underpinnings of tools like GPT-4 or Stable Diffusion were (for the most part) available five years ago. It was, however, the enormous scale of data and compute4 poured into these tools that brought them into existence, enabling AI companies to reap immense rewards. And these rewards do not seem to be slowing down—training larger models with more data continues to lead to performance improvements, defying the expectations set by analysts, economists, and even AI experts themselves.
AI’s production function
Understanding the broader implications of this phenomenon requires understanding the relationship between scale and model behavior. In economists' parlance, we need to know the "production function" of AI: the relationship between economic inputs (like labor and capital) invested into an AI system and economic outputs (like quantity of goods produced) driven by the system.
In a typical production function (like the one visualized below), diminishing returns naturally mitigate market concentration. That is, as a single company expends more resources on its product, the improvement in output (e.g. number of products) typically decreases. Think of a toy factory: early on, hiring more workers allows the factory to produce more toys. But as the factory gets bigger, the floor gets more cluttered and the company starts needing to instate bureaucracy to keep track of the workers—eventually, hiring additional workers does not help the factory’s bottom line at all, and the production function “flattens out.”
So far, these diminishing returns have not emerged in the context of AI systems. Instead, extraordinarily large-scale bets by AI companies have been paying off–improving system performance and ensuring new capabilities—incentivizing developers to continue pushing the boundaries of scale. In terms of the production function, all we’ve been able to observe so far is this:
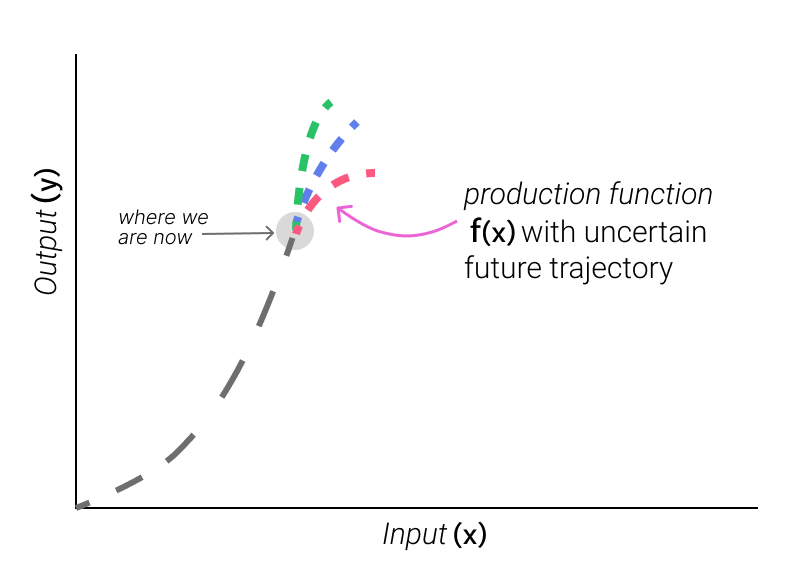
And we have no idea if the future looks like this…
…or like this:
Investing resources according to any of these future curves is a risky, expensive gamble, and one that big AI companies (already operating at the tip of the existing curve) are by far best equipped to tackle.
In other words, playing the scaling game may only be possible for a few players with both significant capital and the conviction that scale will continue to lead to better performance.
Note that market concentration here does not require AI to continue to scale infinitely, or to never see diminishing returns. In fact, we aren’t even ruling out a scenario where diminishing returns have already started. Instead, the issue is that we simply do not know how AI will continue to scale from an economic perspective. Because of the uncertainty surrounding the AI production function, new players will (rightfully) be hesitant to enter the space, likely further entrenching existing advantages around data and computing power.
Data and compute
A common argument against market concentration appeals to fundamental limits around data and computing power—e.g., that there’s only so much data on the internet, and as a result, the production functions above are bound to “flatten out” (and, given the estimated size of modern training sets, do so soon). However, recent developments have challenged this presumption. Advances in speech-to-text will allow large language models to leverage audio and video data; the emergence of multi-modal models such as GPT-4 and BLIP open the door to massive new data sources; and the quality of synthetic data (i.e., fake data generated only to train models) is improving at an impressive pace5.
In fact, the importance of data and compute suggests that existing moats around both might make market concentration more likely. Google and Meta for example (and perhaps now OpenAI, given its recent wave of hiring data-generating contractors) have swaths of private data that they’ve collected from other products. Similarly, cloud compute providers like Amazon (through AWS), Microsoft (through Azure), and Google (through GCP) are also naturally positioned to explore the frontier of AI scaling, having accrued years of high-performance computing equipment and expertise.
Driver II: Data network effects
A second potential source of market concentration in the upstream of the AI supply chain is the presence of data network effects: a self-perpetuating cycle whereby platforms with more users accrue more training data, which in turn fuels better models, thus drawing more users.
Why do data network effects matter?
Generally, a network effect refers to when the value of a product, platform, or service is dependent on the number of people leveraging it. Social media platforms provide good examples of network effects: if all your friends join Instagram, the platform becomes more attractive to you—even if it hasn’t changed or innovated at all. Data network effects are a special instance of network effects that arise because a system learns from the data collected about its users6: the more people use a product, the more data they provide; this additional data helps the product improve (e.g. better recommendations, more accurate classification, or more natural chat experiences). This cycle continues and over time competitors are unable to serve users as well.
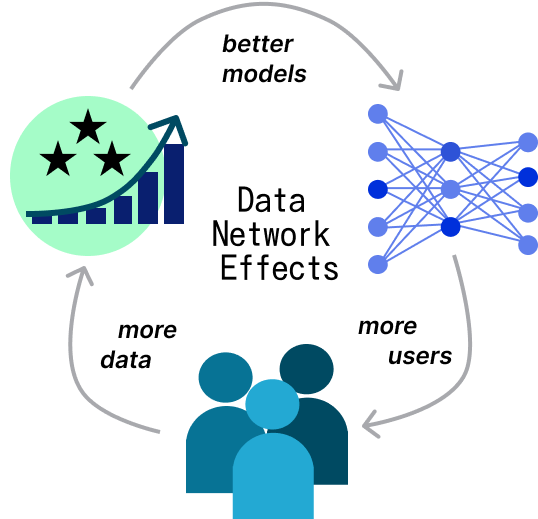
Such a feedback loop can unleash “winner-take-all” dynamics resulting in one or very few companies dominating a market.
Will AI supply chains be shaped by data network effects?
There are two main conditions for base models to exhibit data network effects strong enough to drive concentration:
The system should learn from data gathered through interactions with users
The performance improvement gained from this learning should become so quickly apparent that existing and new users continue to be attracted to the system.
Once these two conditions are met, data network effects will emerge: more users will lead to better performance, in turn attracting further users, and so on.
Early base models (e.g., the very first LLMs) did not satisfy either of these conditions. They were trained on only historical data like Wikipedia or archived versions of pages on the internet. The inaccessibility of base models to the general public also made it difficult to obtain meaningful user data. Moreover, model improvements came from re-training the model on new external data, and were fairly infrequent.
Now, however, the landscape is changing. As more people start to interact with base models, companies can collect useful information, like how users rate a ChatGPT output or whether a user edits an email reply suggested by Gmail. Furthermore, newly-developed techniques like “reinforcement learning from human feedback” (RLHF) enable developers to adapt models more rapidly to this user data.
For now, as far as we can tell, companies are not improving base models in real time based on user data. This may be because companies (rightfully) fear the relatively new method will be prone to manipulation and abuse (e.g., users might try to coerce ChatGPT into outputting racist content by “upvoting” and “downvoting” corresponding answers). Instead, updates to popular base models like ChatGPT and Bard have come every few weeks, which—although rapid compared to previous generations of base models—may not yet be fast enough to trigger data network effects.
And the status quo may already be changing, given cases like the newly released RLHF chatbot from Stability AI. The growing availability of useful user interaction data, coupled with pressures from an increasingly competitive landscape, may provide the necessary incentives for higher frequency model updates and real-time user data integration, making data network effects–and the resulting feedback loop that leads to market concentration–a real possibility.
In summary, although the two conditions for data network effects to drive market concentration do not appear to have been met by current base models, this could change very soon.
Driver III: (Innovation) Platform Effects
Finally, another potential driver of concentration in the AI ecosystem is so-called platform effects (referred to in economics literature as innovation platform effects7), where downstream developers of AI are “locked in”—implicitly or explicitly—to a given upstream AI supplier.
What are platform effects?
Platform effects are widespread across the technology sector. Apple’s iPhone, for example, benefits from platform effects through its tightly-integrated operating system iOS. Features such as one-click sharing or in-app purchases create incentives for app developers to innovate on features that adapt to the idiosyncrasies of both iOS and other existing apps in the iOS ecosystem. These incentives drive innovation platform effects: developers prefer to stay in a platform because of the synergies they find when interacting with the platform and with other applications also developed in the same platform8.
Importantly, not every platform induces the same extent of platform effects. The strongest platform effects emerge when there are incentives to create complementarities, i.e., applications that work together seamlessly because of (and through) the platform.
Will AI supply chains see platform effects?
To what extent do we have to worry about platform effects in AI? Well, the recent wave of AI systems are platforms—they provide a common resource (a base model) on which others can innovate. Given the new announcement of Amazon Bedrock and recent rollouts of ChatGPT plugins, platform effects are likely to soon emerge. The question, then, is whether users of upstream models will create new complementary products and services, adapting to the idiosyncrasies of other products and the upstream models themselves.
There is potential for complementarity among the downstream uses of AI base models stemming from some of the attributes of AI systems. For example, as we discussed in our previous post, AI systems tend to be non-modular and underspecified. Such attributes imply that downstream AI systems may need to adapt to the idiosyncrasies of the base model on which they are built (and that “transplanting” an application to a competing model may be costly). It is then possible that applications developed to deal with and exploit the idiosyncrasies of the same base model end up showing some degree of complementarity among themselves. If such complementaries are relevant enough (and if the right business strategy is adopted), it would support a future where upstream base model providers become (innovation) platforms.
In summary, our analysis of platform effects leads us to the same conclusion as our analysis of data network effects. Even if the effects themselves are not yet fully realized, they have the potential to emerge, laying the groundwork for market concentration.
Takeaways
Pioneers in new industries often strive to maintain their edge through a combination of business strategies such as safeguarding trade secrets, attracting and retaining top talent, or securing preferential access to key resources. These moats are already visible among base model developers at the top of the AI supply chain. OpenAI’s unwillingness to share technical details about GPT-4 is an example of this. However, business strategies alone typically don't result in lasting market concentration. Indeed, early market leaders OpenAI and Google are learning by experience that talent can migrate elsewhere (with competitors Cohere, Anthropic, and Adept founded by their former employees), and trade secrets can't be flawlessly protected9. While effective business strategies can lead to success and profitability, they alone do not result in sustainable market concentration. As economic history has shown, enduring market concentration is primarily driven by technology10.
It is too early to tell if the supply of base AI models will be highly competitive or concentrated by only a few big players. However, the possibility of better-than-expected returns to scale, data network effects, and the innovation platform potential of base model systems make it hard to rule out a concentration scenario. And what of its consequences? Of course, developers of downstream AI applications would bear the brunt of upstream concentration by having a very asymmetric relationship with their main supplier—a topic we'll explore further in our next post. But, also, the far-reaching influence of powerful upstream AI developers may span industries and nations and give rise to unparalleled concerns in economic policy, geopolitics, and national security. We thus cannot simply dismiss these scenarios and trust market forces to foster competition, particularly when the technological groundwork for market concentration may already be in place.
Next time, we consider how the downstream plays into these dynamics, and what the consequences are if either competition or concentration in base AI models becomes our reality.
And possibly a general appreciation for Pi by the tech community!
Vertical integration refers to the case where one company combines two or more stages of production normally operated by separate companies.
By compute, we are referring to computing power–e.g. that which is provided by large numbers of GPUs.
There are multiple types of platform effects, innovation platform effects are most relevant to our current discussion.
Note that researchers were already able to fine-tune an open-sourced language model using text generated by an OpenAI model to get an approximate “copy” of ChatGPT.
Other sources of enduring market concentration are anti-competitive practices (which are illegal in the US by virtue of antitrust law) and government-awarded monopolies (as, e.g., with local electric utilities), but these are not (yet) relevant to the context of AI supply chains.