The Diverse Landscape of AI Supply Chains: The AIaaS Supply Chain Dataset
Post 3.5 in our series On AI Deployment (By Aspen Hopkins, Isabella Struckman, Aleksander Madry, and Luis Videgaray)
In our earlier posts, we outlined what AI Supply Chains are, and why AI Supply Chains might be uniquely challenging to govern. We also discussed how future decisions may (or may not) encourage market concentration. Today, we discuss the structure of such supply chains and introduce our AI-as-a-Service (AIaaS) Supply Chain Dataset to facilitate a systematic view of the diverse configurations seen in AI supply chains.
The deployment of base–or foundation–models and their influence on industry has grown substantially over the last few months. Yet it remains unclear the extent to which these systems will be embedded into new or existing products.Â
As we previously discussed, AI supply chains have existed for some time already. Even before large language models (LLMs) such as ChatGPT entered the scene, products like Google Translate could underpin different services (think automatically translating words on a webpage to encourage language acquisition, or to learn a new language while watching Netflix or Youtube). Similarly, a few years ago, Apple introduced libraries allowing iOS developers to easily embed AI tools in their apps, and now many iPhone experiences are powered by Apple’s upstream AI models.
It’s remained largely unclear, however, what kinds of supply chain configurations exist in the current AI ecosystem, making it challenging to implement and evaluate policy efforts in this space.
Fortunately, work like the AI Ecosystems Graphs has started to fill in some of these gaps by describing where base models are being used in larger, corporate settings. But we also need to map these supply chains out on a finer-grain level, going beyond the major players like Coca Cola or OpenAI. After all, long-tail players are an important part of the AI industry ecosystem.Â
To this end, today, we are releasing a dataset that also encompasses less prominent actors, enabling a more comprehensive view of AI supply chains. The dataset includes a wide array of both upstream organizations and products–such as base models–and downstream actors–such as AI start-ups, along with their contribution to the development of AIaaS products. And we’re still adding to it, with the hope that opening it to the public allows us to pull even more organizations into this effort!
If you have additions you'd like to recommended we add to the dataset, please submit them using the following form: https://forms.gle/gsccWsSKpjsg9UKq5
Let’s delve into the dataset’s specifics.
The AIaaS Supply Chain DatasetÂ
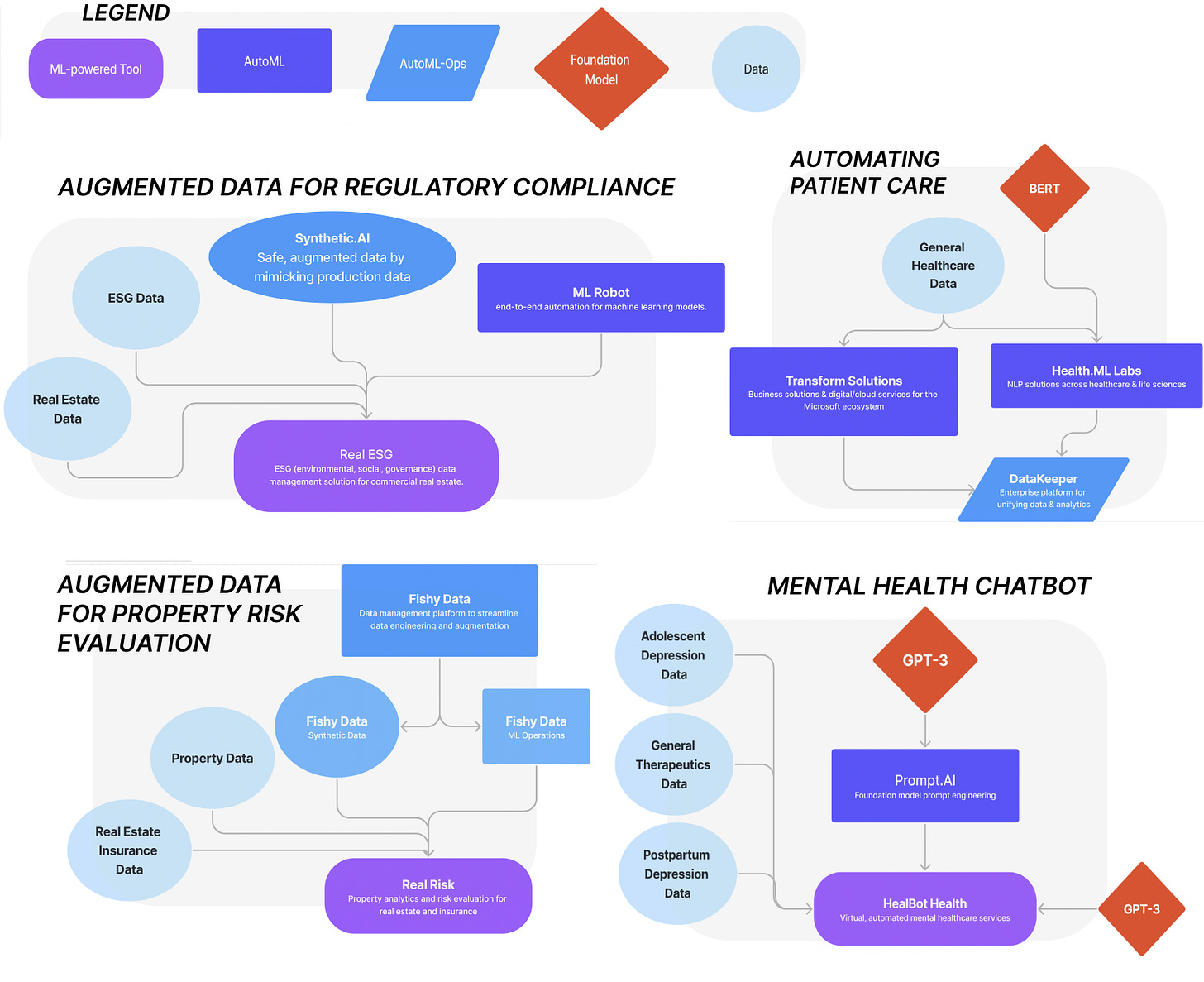
Sourced largely from existing datasets built by venture capital efforts to track the AIaaS industry, the dataset categorizes companies by their contribution to the learning process of ML-powered tools. It includes AIaaS positions in the stack, categories, subcategories, industry-specific labels, company descriptions, and, of course, URLs:
AIaaS Stack Position: Company's position within a (four-tier) AIaaS stack: Infrastructure, Machine-Learning Operations (MLOps), Automated Machine Learning (AutoML), and ML-Powered Tools (descriptions of each can be found in this git repository).Â
Category: Â The type of product or services the company provides. Should offer further insight into the company's position in the AI supply chain.
Industry Labels (optional): Assigned to companies that are focused on catering to a particular non-AI industry (e.g., healthcare, finance, or legal).Â
Subcategory: Additional details or keywords related to the company's offerings. This information is useful for quickly differentiating companies in similar roles within the ecosystem.
Descriptions: Longer summaries of company services, built with a combination of web scraping and manual data entry.
Users can access the dataset using the git repo, or by accessing a Google Sheet. For more information on the dataset or how to use it, please see the Github readMe page.Â
We’re excited to see how this dataset develops. Expect our future posts to pull on some specific examples from this dataset!Â
Check out our dataset here.
In our next post, we discuss the landscape of downstream actors in AI supply chains. What should we be paying attention to? What might we be missing?