Downstream AI products benefit (and suffer) from access to upstream AI
The fourth post in our series On AI Deployment (By Sarah H. Cen, Aspen Hopkins, Andrew Ilyas, Isabella Struckman, and Luis Videgaray)
In our previous post, we discussed potential sources of market concentration in the upstream layers of AI supply chains and determined that we cannot yet rule out the possibility of a monopoly or oligopoly in upstream AI (where base models reside). In this post, we examine the market structures likely to arise in the midstream and downstream layers of AI supply chains and consider how upstream market concentration might affect the burgeoning AI ecosystem. Read our series for more on AI supply chains.
In 2023, AI is everywhere. Up until now, most of the public’s attention has been on recent advances in base models, like GPT-4 and Stable Diffusion. But base models are only part of a much larger, more complex AI ecosystem. So, what else should we be paying attention to?
In this post, we’ll argue that much of the explosive development in AI is actually happening farther along the AI supply chain1. In an AI supply chain, base models and datasets constitute “upstream” AI: they supply goods and services that are used by other companies. These other companies—that utilize upstream AI for specific domain applications—make up what we call the “midstream” and “downstream” layers of the AI supply chain.
The surge in midstream and downstream AI products matters. As companies rush to harness the capabilities of powerful, general-purpose base models and datasets for their own purposes, the AI supply chain will continue to expand. Although there will almost certainly be growth at midstream and downstream layers, the situation might be different upstream. In this post, we’ll explore the implications of AI supply chain growth and, in particular, what may happen if the number of upstream players remains stagnant.
What do we mean by “Downstream” AI?
Before we unpack the dynamics between upstream and downstream AI, let’s define what we mean by “upstream,” “midstream,” and “downstream” AI.
While every component in the AI supply chain offers goods and services, there are some components that are higher—or more upstream—in the supply chain. These include base (or foundation) models—like OpenAI’s GPT-4, Anthropic’s Claude, and Google’s PaLM 2—as well as large datasets on which base models are trained. Generally speaking, upstream components have the key characteristic that they can be repurposed in many different ways.
Farther down the AI supply chain are components that we refer to as downstream AI. These components are typically products that directly interface with users, such as an AI-driven hiring tool or shopping app. There are, in addition, many components in between that we refer to as midstream AI. A hiring tool, for example, may be built on top of a midstream AI model that summarizes resumés. Or a shopping app may solicit a customer’s opinions on a midstream dataset of AI-generated outfits2. For a primer on AI supply chains, see our second post.
The relationship between upstream, midstream, and downstream AI is the focus of this post. While most of the public’s attention has been about upstream AI (like GPT-4), we want to discuss what will happen midstream and, in particular, downstream.
Downstream AI is becoming easier to develop
AI supply chains are making AI more accessible, so much so that AI-driven products will soon become ubiquitous. This is partially due to what’s now emerging at the upstream layers. AI has previously been difficult to harness, requiring significant resources as well as expertise. However, the rise of base (upstream) models and datasets drastically reduced the cost of AI development and lowered barriers to entry. Upstream components are now doing a lot of the leg work for us. Base models are trained in a general-purpose way that allows downstream models to learn new concepts with significantly less data and compute. Similarly, base datasets provide a raw material that would be expensive to curate from scratch.
Midstream components make upstream AI even more accessible. They help to bridge the advanced capabilities of upstream models with the specific, practical applications found downstream. For example, a hiring tool may wish to use midstream models that perform intermediate tasks (like summarizing resumés or generating interview questions) rather than figure out how to use GPT-4 for the same purposes (which might require additional expertise, like prompt engineering).
Together, these factors have made it increasingly easy to develop downstream AI. People can now harness the power of AI without any technical training, and the cost of developing an AI-driven product has dropped dramatically. So, what’s next?
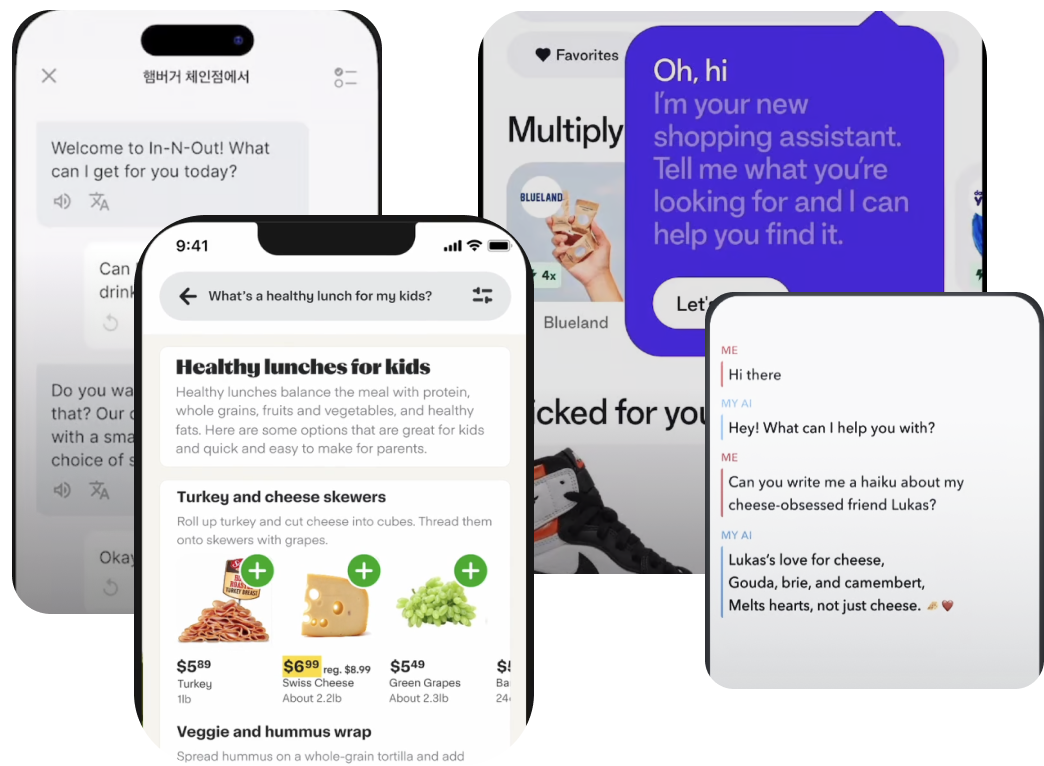
AI supply chains have a strong gravitational pull
As AI becomes easier, cheaper, and faster to harness, we will see an explosion of AI-driven products. Think of an insurance company that is limited by its ability to correctly process claims or a law firm whose main time sink is bookkeeping and administrative tasks. AI-driven software can automate many of these processes, increasing productivity and driving creativity in a way that seemed like science-fiction not too long ago.
The observation that AI will drive productivity and creativity across various industries isn’t new. What’s particularly noteworthy is that these AI-driven products will likely emerge from an ever-growing AI supply chain. That is, the vast majority of AI-driven products won’t be developed independently—they’ll be built on top of a long chain of AI components.
Why? For one, the rise of base models and datasets presents AI companies with a trade-off.
Barriers that previously prevented startups from succeeding will be reduced with the rise of the AI supply chain–this means that AI is leveling the playing field, allowing startups to thrive in greater numbers than before. These organizations must either invest in expensive in-house AI development or join the AI supply chain and build their products on top of upstream AI components. Many will choose the latter option, seizing the low-cost opportunities offered by the AI supply chain.
For another, new quality standards will emerge as competitors incorporate upstream AI models into their products. These standards will incentivize outsiders to opt into the AI supply chain rather than develop in-house models (as these are likely to have poorer performance). Companies that resist joining the AI supply chain will be compelled to invest in AI to keep up with the rising standards of goods and services.
The AI supply chain thus has a strong gravitational pull, leading it to expand and grow along the mid and downstream layers.
Dependence on upstream AI has downstream consequences
It’s clear that the AI supply chain will grow at the midstream and downstream layers. As for the upstream layers, there are two possibilities: competition or concentration. We must pay careful attention to these two possible worlds because the AI supply chain creates what we call a “dependency”—countless midstream and downstream players will rely on the goods and services provided by upstream components.
One possibility is healthy competition. A multiplicity of upstream components—including open-source alternatives—would benefit downstream AI companies for several reasons, including:
Choice of upstream providers. Under healthy competition at the upstream layers, midstream and downstream developers would have more flexibility in what they choose. If one upstream component does not suit their needs for any reason, they could turn to another.
Healthy downstream competition. Upstream competition helps facilitate better downstream competition—more alternatives upstream can lead to more alternatives downstream (if all downstream companies got their models and data from the same sources, there would be little room for downstream companies to differentiate themselves).
End user experience. Upstream competition is also good for users, as competition across the board fosters innovation, higher quality products, and lower prices3.
The other possibility is upstream concentration. As explained in our previous post, there are technology-driven reasons concentration at the top of the supply chain may occur, including uncertain returns to scale, data network effects, and innovation platforms effects. If such factors result in upstream concentration, then midstream and downstream AI companies may find themselves in a highly asymmetric relationship with their upstream providers.
There are many possible implications of upstream concentration, including:
Economic consequences. Concentration grants upstream AI providers a technological monopoly. While there are a number of economic consequences for the downstream market, three possibilities stand out.
Monopolistic rent extraction. Base model suppliers might rely on unfair pricing strategies to “squeeze” downstream providers’ profits, ensuring they fully capture the “rent” that their monopolistic power creates.
Choosing winners. With power asymmetries, providers may give preferential treatment to select customers, raising the barrier to entry and reducing competition downstream.
Selective vertical integration. As upstream AI providers grow, they may build competitors to existing verticals, forcing out downstream competitors.
Performance consequences. Beyond economics, there are technical implications of upstream concentration for downstream AI. First, redundancy is decreased—base models frequently experience unexpected or undocumented updates that developers must account for to maintain their product’s performance. There are even instances of providers discontinuing base model services (see Codex). If there are only a few upstream providers, the effects of performance degradation will propagate to end users. Further, downstream developers currently must adapt their products to the idiosyncrasies of a single upstream model. Any modifications to an upstream model can lead to product malfunctions or unexpected behaviors that are challenging to manage.
Policy consequences. Finally, there are consequences for AI governance. For example, power asymmetries enable upstream providers to allocate liability downstream (e.g., through non-negotiable terms of service). The existing (and largely undiscussed) challenges of uncovering liability when AI is deployed through complex supply chains further encourages this outcome. Other issues such as algorithmic monoculture and bias transfer also arise from upstream concentration, and might require dedicated policy solutions.
Generally, monopolistic practices lead to negative impacts on the availability and cost of AI capabilities that originate at the top of the supply chain. Although the emergence of large base models and datasets comes with the promise of making AI easier, faster, and cheaper, upstream market concentration would (at least partially) undermine the benefits of this promise.
What we don’t know
As we discussed in our prior posts, we don’t yet know what the future holds. The case has been made that developers of base models (including Google and OpenAI) do not have the technological moats to protect themselves from open-source competition (although it is unclear if this trend can continue once upstream developers stop sharing their models with the open source community). We do know, however, that market concentration upstream will have significant downstream effects.
It’s similarly too early to tell if downstream AI markets could experience concentration in the long term. If downstream concentration does occur, we don’t think the driving factors will be introduced by the AI supply chain but rather from existing, non-technical factors of existing market ownership reinforcing highly profitable or defensible positions (think domain-specific data or exclusive relationships with valuable clients).
Instead, we expect competition at the mid and downstream levels of AI supply chains will be most influenced by the decisions that companies and policy makers are making right now to shape the upstream market. If steps are taken to mitigate upstream concentration, competition at the mid and downstream layers of AI supply chains will be maintained.
Where we go from here
For entrepreneurs and investors, the current state of AI means new business models over-relying on the easy availability and low cost of upstream AI may not be sustainable. For policy makers and regulators around the world, this should translate to a call to action to closely monitor pricing, liability allocation, technical updates, and preferential treatment practices of upstream AI providers (and for antitrust authorities to be rather skeptical about vertical integration acquisitions in AI).
In our next post, we will explore concrete AI policy ideas based on our discussions throughout this series.
In one of our previous posts, we introduced the notion of a complex “AI supply chain” as the prevalent form of AI deployment into the world. That is, most of the AI systems that we interact with are typically a product of many AI components glued together, therefore dividing AI companies into different layers of the AI supply chain. Moreover, in our latest installment, we published a database that illustrates the current complexity of the AI supply chain.
An AI component does not need to fall neatly into one of the three categories. Take ChatGPT, for example. (For a primer on AI supply chains, see our second post.)
Even if the upstream exhibits harmful concentration, users would still benefit from competition at the downstream levels by avoiding the “double marginalization” (or double monopoly markup) problem, in which the price to the user is even higher than the price that a vertically integrated monopoly would charge (in short two monopolies across a supply chain are worse than only one). Tirole (1988), Staal Gabrielsen et al (2018)