Three proposals for regulating AI
Our fifth post On AI Deployment (By Sarah H. Cen, Aspen Hopkins, Isabella Struckman, and Luis Videgaray)
In our last few posts, we discussed how the growth of AI supply chains affects AI developers and consumers. We considered how consumers and mid/downstream developers are especially at risk if upstream providers (e.g. of base models) maintain asymmetric power over the downstream. Yet the current framing of AI policy does little to de-risk this scenario. In today’s post, we highlight three factors that are critical to a healthy AI ecosystem—(1) fostering competition, (2) allocating liability, and (3) standardizing disclosures—and outline how we might design policies that account for these two considerations.
AI supply chains are quickly growing ubiquitous. Upstream providers are introducing models and datasets that are increasing access to AI—a technology that historically required great expense and expertise. Although the complex systems powering AI supply chains are poorly understood, there are several concrete and persistent concerns we must be aware of:
First, upstream developers may gain substantial market power, and there are a multitude of ways that this power might be abused. We outline several in our prior post.
Second, AI products deployed through AI supply chains are difficult to audit or explain. See our second post for more information.
Finally, who bears liability in an AI supply chain is currently unclear. As a result, responsibility may be shifted down the supply chain, leaving upstream players unaffected.
Today we’ll argue that there are three aspects of the AI industry where early intervention is both possible and helpful for ensuring sustainable growth: fostering competition, allocating liability, and standardizing disclosures. Out of the many potentially impactful steps that policymakers might take, targeting these three factors is both tractable—meaning a solution is possible—and conceptually simple, with a diverse spread of existing regulations across other industries to take inspiration from.
Our discussion of what to regulate (competition, liability, and disclosures) further considers who to regulate (it’s complicated, but upstream providers at the very least) such that we best respond to the concerns we list above.
Let’s discuss.
What to regulate
Regulating a rapidly changing technology that we don’t fully understand is challenging. While we aren’t certain what an ideal future for AI is, society does have clarity on what we hope to prevent, including violations of personal privacy, unexplainable decisions, and inequitable outcomes. Yet we struggle to mitigate these issues even within a single AI system. As a result (and accounting for differences in cultural and economic priorities), AI policy is internationally quite disparate.
On one hand, the EU has invoked a number of requirements regarding transparency, copyright, and privacy. Italy temporarily banned ChatGPT (citing concerns of privacy and underage access to inappropriate material). In contrast, Japan’s focus has been on supporting the budding industry, going so far as to propose removing copyright restrictions for material used to train AI models. China has proposed targeted regulations emphasizing requirements for truthfulness and accuracy, while the UK is expanding regulations on human rights, health and safety, and competition rather than creating a new regulatory body. Most policy makers agree that both cultivating and moderating this evolving industry is critical, but threading the needle remains a challenge. How can policy help in scenarios where products are deployed via multiple complex AI components?
Today, we argue that there are three topics that policymakers should focus on: fostering competition, allocating liability, and standardizing disclosures.
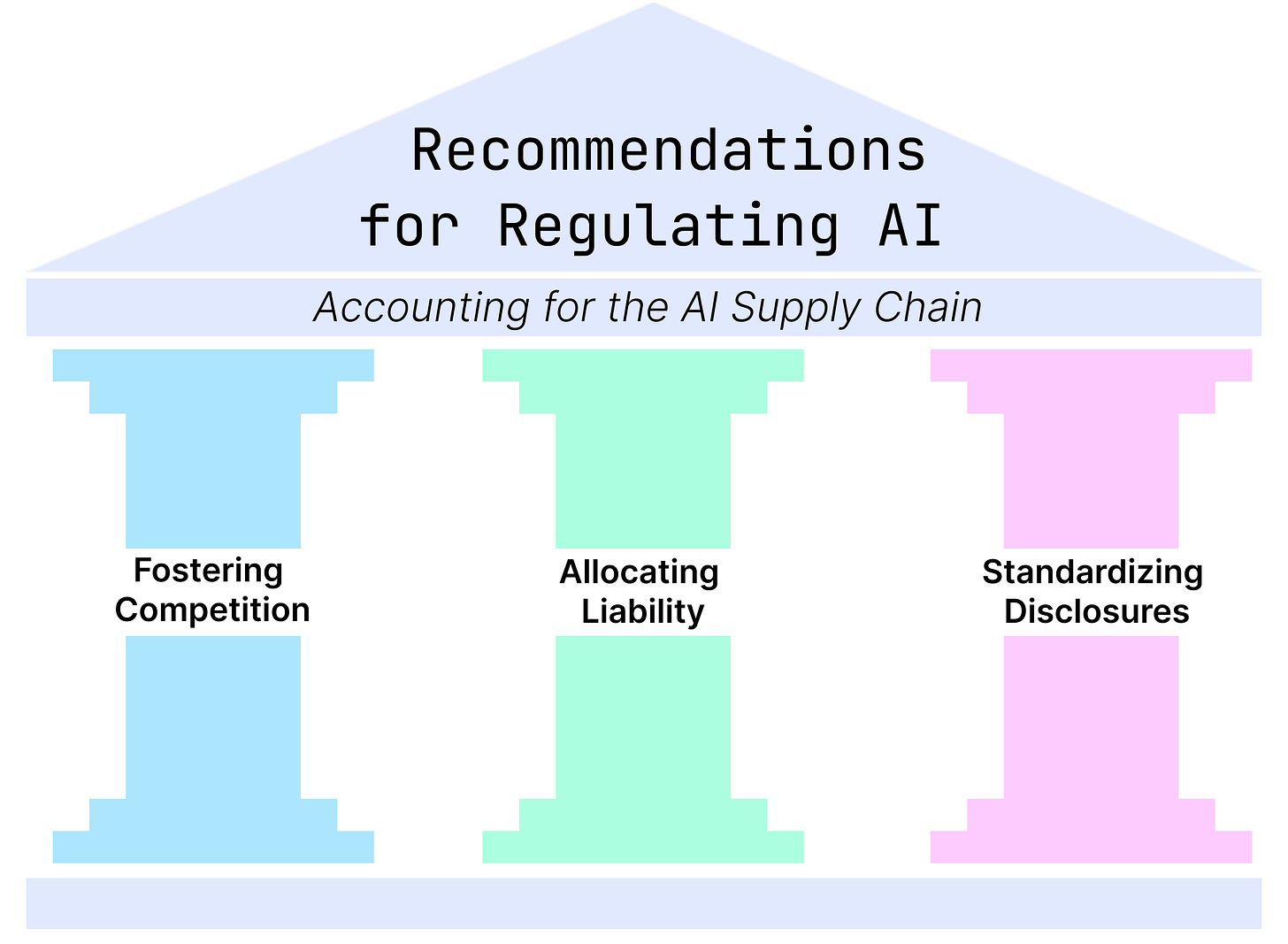
Fostering competition
In our previous posts (here and here), we considered how healthy competition in upstream providers would shape the AI industry and how the alternative (market concentration) could harm an otherwise robust mid- and downstream ecosystem. Market concentration produces a climate where upstream providers may choose to wield economic and performance pressures indiscriminately. These power dynamics make it difficult for midstream and downstream users to challenge the status quo, whether that’s in regards to unfair pricing, uneven distribution of liability, or requesting transparency in upstream product updates. Market concentration also exacerbates the question of what values AI models should uphold. If only one language model is dominant, for example, all subsequent downstream models will be shaped in its image, reflecting a limited set of “beliefs”.
On each of these fronts, competition can help. Currently, consumers and mid/downstream developers are limited by their options. In many cases, it’s impossible to compete with the quality of products companies like OpenAI, Google, Anthropic and others are offering. In the long-term, this means opting into a set of values or practices that only a few key players are able to shape. Instead, a robust industry is one where there are multiple options to choose from, particularly upstream. We believe policymakers can support competition in three ways.
Subsidizing access to computing for small businesses. At the moment, compute (which is necessary for model training and development) is expensive.Moreover, it is not always allocated fairly across those who need it (for instance, priority is often given to large corporations). Although there are efforts to fix this, subsidizing compute from cloud providers (like AWS) for small businesses serves to benefit the supply chain as a whole.
Incentivizing the production of open-source models and datasets, which would allow independent developers to use and modify existing code rather than start from scratch.
Subsidizing data marketplaces, subject to strict terms of use. Data marketplaces allow individuals and businesses to buy and sell data. When they are executed responsibly (e.g., while protecting user privacy), data marketplaces have several benefits. For one, individuals selling their data not only have more say over who gets their data, but are also compensated for their data. Importantly, such marketplaces can allow small businesses to source data—a resource that, for the most part, falls in the hands of tech giants like Meta and Google.
Allocating liability
Simply put, product liability1 determines what party is held legally responsible when a failure or defect occurs in an item, allowing those impacted to seek recompense. Modern laws regarding product liability extend purview outside of physical (tangible) consumer products to include intangibles such as gas, naturals (i.e. pets), and even writing (i.e. navigational charts).
Product liability2 makes it possible for any party within a supply chain to be held liable, from the manufacturer of a product, manufacturers of its individual components, product assemblers, or even the wholesaler or the retailer. In exchange, affected parties must prove negligence or wrongdoing occurred.
This is where AI introduces a unique challenge. As the 2022 EU AI Liability Proposal motions to, characteristics of AI make it challenging to identify liable parties and gather the proof needed for a successful liability claim. As a result, new bodies of AI policy seem to emphasize risk prevention and management, accompanied by a favorable attitude towards potential claimants in the event of damage.
Without careful attention, this means the burden of responsibility will often fall on the “last mile”—the organization that interfaces most immediately with consumers. This allocation of responsibility is further enabled by stringent terms of service by upstream players (e.g., those that provide base/foundation models). But consumer protection and antitrust authorities have an opportunity to change this before it becomes established practice. In particular, regulators with pro-competition purview (such as the US Federal Trade Commission) can proactively use their existing authority to prevent one-sided terms of service which fully shift liability downstream. To do so effectively, policymakers must also ensure that developers—particularly, midstream and downstream players—are aware of the risks and responsibilities that they take on, as we discuss next.
Standardizing disclosures
To complement these regulatory steps, standardizing disclosures can protect the interests of both upstream providers and mid/downstream developers. Disclosures involve distributing information—including negative details—about products, corporations, individuals, investors, and legal cases to all involved parties to ensure a common set of facts are used during a decision-making process (for example, public companies typically disclose financial data to investors). Disclosures are common across industries and include, for instance, nutrition facts, warning labels, and product specifications.
More concretely, in a scenario where liability is shifted down the AI supply chain, providing appropriate context (such as when a model or dataset is updated) protects foundation/base model providers while informing mid/downstream development.
There’s precedent for such a move. AI fairness research has long espoused the value of documentation to calibrate dataset and model use.3 Metadata has become relatively common, particularly in the public sector4, to describe how data was collected along with various facets of relevant information. And disclosures, the mode through which many industries communicate information relevant to a given scenario, are an integral aspect of day-to-day efforts. The challenge isn’t in determining if disclosures should be incorporated into AI compliance requirements, it’s how.
Disclosures should provide protection to all participating parties, but there are many modes in which they might be constructed. As a starting point, we can borrow elements from the ways that they are applied across various domains.
Disclosures should maintain consistency in structure, legal requirements and language. Such consistency means that people know what to expect, what to produce, and naturally allows for easier auditing. Consistency minimizes uncertainty for all parties—a win-win.
Disclosures must balance the act of informing users with oversharing (proprietary information), prioritizing safety throughout. To support midstream and downstream developers, and of course consumers, upstream providers should be transparent about what they know about the performance and risks of their own base models (including what they don’t know). Midstream and downstream developers might similarly be asked to share context about application dependencies.
Finally, with the introduction of AI supply chains, disclosures must also account for the interactions between various layers of AI supply chains. This is entangled with the above recommendations, as it is yet unclear what information upstream and lower layers should have a right to.
We frame pros and cons of placing disclosure requirements on different aspects of AI supply chains below.
Upstream disclosures
The idea of upstream players informing mid/downstream users (e.g. through disclosures) isn’t particularly surprising. The EU’s proposed AI Act acknowledges this by requiring providers include performance guarantees for their models, though it’s unclear what such guarantees entail. Given the state of the AI ecosystem, disclosures should include information about model performance, various dataset and training characteristics, and perhaps even performance on a set of known, published benchmarks to give approximate details that can later be used to calibrate downstream expectations.
Further, disclosures could account for any modifications to a model or dataset which influence the model’s behavior. If updates to models are rolled out once a week, then there should be a notification of that change, along with a detailed comparison between previous and updated performance. Further, upstream providers should enable access to older versions of models for some set period of time to allow downstream participants the time needed to robustly evaluate changes and transition over. These steps are critical because developers and users build expectations of model performance. Without appropriate notification, discrepancies between expectations and reality can lead to harmful (and avoidable) outcomes.
Midstream and downstream disclosures
While it’s clear that upstream providers should inform those that use their products, it’s unclear if the reverse should also occur. Should mid/downstream organizations provide disclosures to upstream providers? Should such disclosures be held to a similar level of transparency and stringency? These questions remain relatively unexplored but warrant consideration.
If disclosures act as a two-way mode of communication (where both upstream and mid/downstream organizations share salient information), then they may be doubly protective of consumers. For example, upstream providers may ask for assurances that the downstream takes appropriate precautions ensuring equitable products.5 This reduces the risk of litigation across the entire supply chain. And, in a world where upstream market concentration may become the norm, asking mid/downstream developers to provide context upstream enables easier auditing for only a handful of companies. As a result, we might expect these upstream providers to hold their contracts accountable to some standard of safety (whether in issuing recommendations and warnings, or taking corrective actions if needed).
The challenge with this formulation is that providing information to upstream providers may unintentionally encourage vertical integration or put an unfair burden on resource-constrained organizations. While neither scenario is desirable, with careful consideration we might be able to thread the needle, encouraging transparency while helping to balance liability across the AI supply chain.
A side note on midstream organizations. For most of our prior posts, we’ve combined the midstream and downstream. This is because the midstream shares the downstream’s burden of being dependent on the beneficence of upstream providers. But in the case of liability, midstream products may also face many of the concerns indicated for the upstream. How this plays out in the long term is unclear, but lawmakers should be aware of this potential conflict in status moving forward.
Looking forward: how to regulate
Our comments today focus on competition, liability, and disclosures. We expect that the regulation of each will depend on the domain. Moving forward, AI will largely be governed by legislation that existed before AI rose to prominence and through existing domain-specific regulatory bodies (FDA, FTC, SEC, etc). However, there are gaps that surface when adapting existing regulations and their governing agencies to AI systems, especially in the presence of complex AI supply chains. The three policy directions we introduce in this post compensate for these gaps, supporting the burgeoning AI industry while complementing existing regulations designed to ensure AI models are developed and deployed safely. While regulating AI is an enormous task, failure to consider the complexities of AI—particularly through the lens of the AI supply chain—will challenge an otherwise robust AI ecosystem, and more importantly, harm consumers.
This is just one of several types of liability, though most appropriate for the topic of AI. The EU’s AI Act similarly aligns definitions of liability in AI to product liability. See the AI Act Exploratory Memorandum for more details.
Products liability can fall under negligence, but is generally associated with strict liability, meaning that defendants can be held liable regardless of their intent or knowledge.
For popular examples of model and dataset documentation, see Mitchel et al (2020)’s Model Cards and Gebru et al (2021)’s Datasheets for Datasets.
See New York City’s Metadata For All Guide as an example of municipal implementation of metadata to inform data users of relevant context.
Note that we are not referring to consumers but to businesses and organizations actively participating in the AI supply chain.